Table Of Content
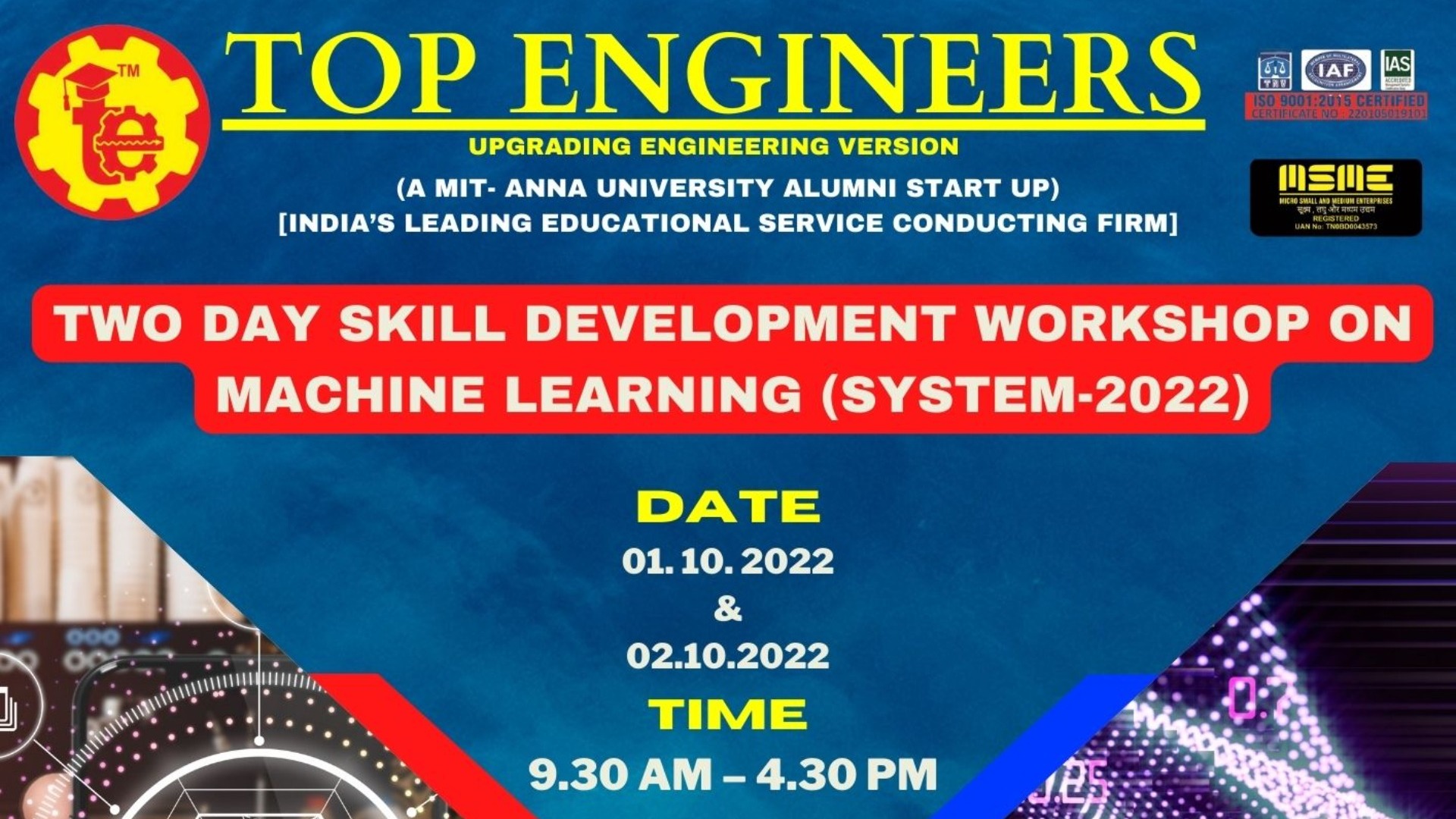
As I mentioned in my first article, I think of systems design questions as improv presentations. The interviewer gives you a task, you clarify it and then present a solution. It’s crucial to go into the interview with a game plan for discussing your design. There’s a lot of ground to cover in creating an ML system and you also need to show some real depth in a few areas. Here’s a flow for an ML question, in reality, it’s easy to blend these topics at any time or take a deep dive. Make sure you look for clues from the interviewer that they want to hear more about a topic, or if you’ve covered enough and you can move on.
Mock interviews
Iterating through multiple approaches doesn’t lend itself to every problem though, sometimes there’s one well known high level approach. I think it’s best to stick with well known industry patterns, there’s room for creativity in the application details. This is also where it helps to be aware of common ML/AI solutions in industry. This book is not a replacement to machine learning textbooks nor a shortcut to game the interviews.
book.json
For instance, a machine learning model based on racially biased data will simply learn to automate racial bias. Even the most performant algorithms are useless if they are not based on quality dataset. As a candidate, I’ve been interviewed at a dozen big companies and startups. I’ve got offers for machine learning roles at companies including Google, NVIDIA, Snap, Netflix, Primer AI, and Snorkel AI.
How to get hired as a Machine Learning Engineer - Towards Data Science
How to get hired as a Machine Learning Engineer.
Posted: Sat, 15 Feb 2020 17:34:10 GMT [source]
Model Development
You’ll walk step-by-step through solving these problems, focusing in particular on how to design machine learning systems rather than just answering trivia-style questions. If you have a machine learning or system design interview coming up, you’ll find the course tremendously valuable. Top tech companies ask system design interview questions to see if you can efficiently solve real-world problems. Today we’ll discuss how you can ace machine learning interviews using system design concepts. I’ve also consulted several startups on their machine learning hiring pipelines. Hiring for machine learning roles turned out to be pretty difficult when you don’t already have a strong in-house machine learning team and process to help you evaluate candidates.

Nobody knows everything though, it’s perfectly fine to miss a few random topics. For recommendation systems, nearest neighbours can be very useful, especially if you’ve embedded your candidates into a lower dimensional space where distance represents similarity. For candidate generation, you often want to select the k closest items in a catalog.
No, you won’t be able to run a million high dimensional pictures through a Resnet model in real time. See the Infrastructure Components section below for some important ML infrastructure. Note that this is common for interview loops for ML generalists like myself.
Minimum Viable Study Plan for Machine Learning Interviews
The quality and quantity of training data is a big factor in determining how far you can go in your machine learning optimization task. Data collection techniques primarily involve user interactions, human labelers, or specialized labelers. For the most part, I found that I wasn’t grilled too ‘deeply’ (pun) on deep learning. There are lots of ML positions that don’t touch deep learning. This is a pretty wide list of the topics that may come up during an interview.
Provide feedback
Let’s discuss the thought process required to answer an interviewer’s questions. Our goal is to improve our metrics when working on an ML-based system. We also want to ensure that we meet the capacity and performance Service Level Agreement (SLA). Performance-based SLA ensures that we return results within a given time frame (e.g. 500ms) for 99% of queries. Capacity refers to the load that our system can handle (e.g. the system supports 1000 queries per second). It has made rapid progress in areas like speech understanding, search ranking, and credit card fraud detection.
Data Brainstorming and Feature Engineering
Companies are leveraging these technologies across industries from healthcare and agriculture to manufacturing and retail. This interview question is designed to get signals on how good you are at applying ML/AI to real world applications. Apply the best techniques in order to structure and drive your interview. The end goal is to explore which features are important and get rid of the redundant ones. Unnecessary features tend to create issues in model training usually known as the curse of dimensionality.
These papers show there’s a wide range of ways to create state of the art recommenders, it’s definitely not a cookie cutter problem. Pay attention to the setups, how they do offline and online evaluation. Incorporate feedback on areas to focus on and see if they bring up red flags or criteria you’ve missed. This is also a spot to make sure you’ve fully understood the problem setup, before you get too deep in a solution.
As the use of machine learning in the industry is still pretty new, a lot of companies are still making it up as they go along, which doesn’t make it easier for candidates. As a friend and teacher, I’ve helped many friends and students prepare for their machine learning interviews at big companies and startups. I give them mock interviews and take notes of the process they went through as well as the questions they were asked. A high level of technical skill is required in the machine learning field, particularly for machine learning engineers.
No comments:
Post a Comment